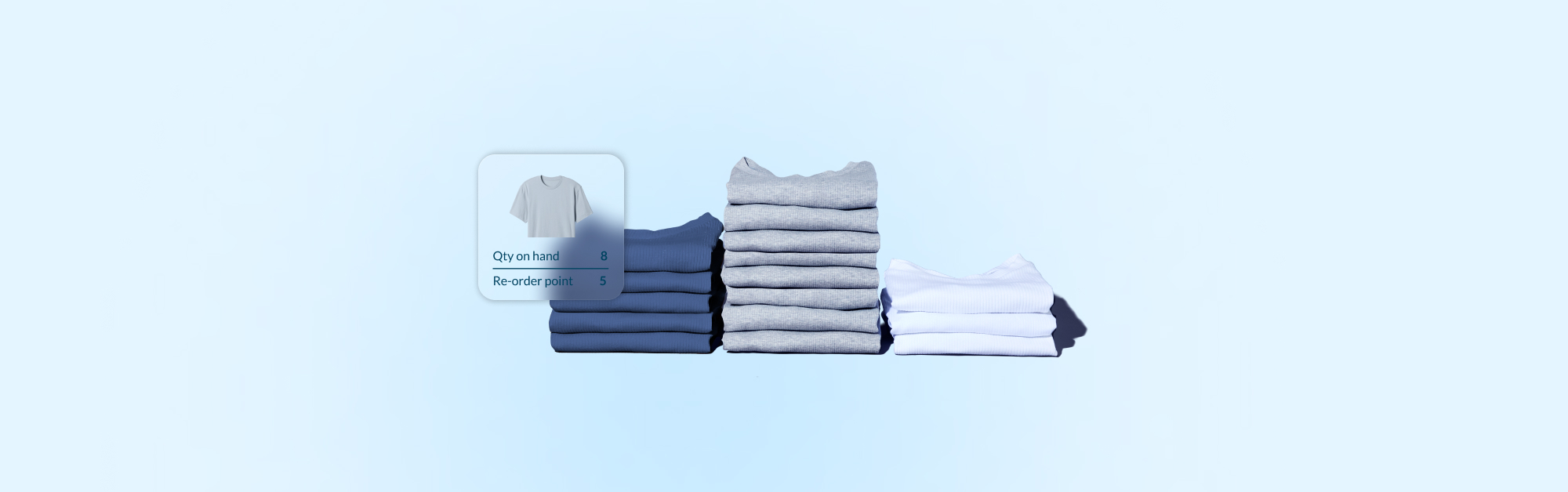
Accurate demand forecasting is vital for any business to reduce costs and meet customer demand.
From qualitative approaches that rely on expert insights to advanced machine learning techniques, demand forecasting methods vary in complexity and application. Retailers and manufacturers alike can use these methods to gain a competitive edge by aligning inventory with market demand, minimizing waste and boosting profitability.
But what are those methods—and when should you use them?
In this blog, we’ll go over:
- What is demand forecasting
- Types of demand forecasting
- Choosing the right forecasting method
- The role of technology in stock management
- Challenges in demand forecasting
The Value of a Unified Payments and POS Solution
An in-depth look at how a streamlined platform for payment processing can transform the way you operate your business, especially compared to legacy systems and patchwork solutions.
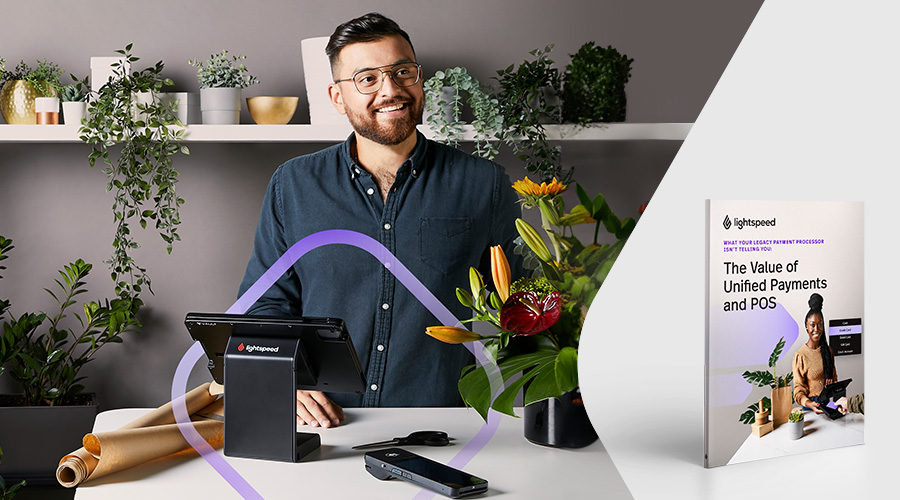
What is demand forecasting?
Demand forecasting is the process of predicting future customer demand for products or services, using historical data, market trends and other variables. It plays a critical role in inventory management and business planning by helping businesses anticipate demand fluctuations, optimize stock levels and reduce costs.
Accurate forecasting ensures that businesses maintain the right amount of stock, preventing issues like overstocking or stockouts, both of which can lead to lost sales and higher costs. Demand forecasting also helps in planning for promotions, seasonal shifts and new product launches, improving operational efficiency.
Types of demand forecasting
Demand forecasting isn’t a one size fits all affair. Depending on your needs, data, industry and market reality, you’ll want different models for different times.
Here are the top four categories of demand forecasting you need to know about.
Forecasting type | Description | Example |
Qualitative | Relies on subjective judgment and trends, useful for new products, markets or when data is lacking. | A retailer planning an eco-friendly line surveys customers to gauge interest. |
Quantitative | Uses mathematical models and historical data to predict future demand, ideal when patterns or trends are apparent. | Time series analysis to anticipate sales spikes during holidays or back-to-school seasons. |
Hybrid | Combines qualitative and quantitative methods to improve accuracy, useful for uncertain or rapidly changing markets. | A retailer launching a new product supplements market research with time series analysis of similar product lines. |
Advanced methods | Machine learning (ML), artificial intelligence (AI), and collaborative planning, forecasting and replenishment (CPFR) offer deeper insights and higher accuracy. | A retailer uses CPFR, collaboration between retailers and suppliers, to align stock levels of a best seller during a high-volume shipping period. |
Qualitative forecasting: for when you have variables without historical data
Qualitative forecasting generally relies on subjective judgment and trends rather than numerical, first-party data, making it highly relevant for retailers who need to anticipate trends or customer preferences in uncertain or rapidly changing markets.
Unlike quantitative methods, which use historical data, qualitative methods are best used when launching new products, entering new markets or dealing with incomplete data.
This often involves doing market research, like collecting data through surveys, focus groups and interviews. For example, a retailer planning to introduce a new line of eco-friendly products may survey customers to gauge their interest in sustainability and understand what features they prioritize. While the retailer in question has no historical sales data to judge how well those products would perform, responses to their survey would give them an idea of if the product line would be a sound investment.
Alongside market research, retailers can use expert judgment in qualitative forecasting, where experienced internal personnel or external industry experts are consulted to forecast trends. A luxury fashion retailer may ask its lead buyers, who have deep market insights, to predict which styles will be in demand next season based on fashion shows and industry events.
Putting it into practice: qualitative forecasting with the Delphi Method
The Delphi Method is a structured qualitative forecasting technique that gathers input from multiple experts in several rounds until consensus is reached. You might use this method when planning for holiday season demand.
Here’s how it’d work:
- Identify experts: select a group of internal or external experts who possess knowledge in relevant areas, such as sales, marketing and operations.
- Initial questionnaire: send a survey or questionnaire to these experts, asking for their initial forecasts or opinions on future demand for specific products or categories.
- Analyze responses: collect and analyze the responses, looking for areas of agreement or divergence in the forecasts.
- Provide feedback: share the summarized results with the experts, allowing them to reconsider their initial opinions based on the collective feedback.
- Repeat the process: conduct several rounds of surveys, refining the forecasts each time. Each round should move closer to a consensus among the experts.
- Reach consensus: after multiple rounds, the final round should produce a forecast that reflects a consensus, which can be used for more informed decision-making in demand planning.
Quantitative forecasting: for analyzing hard data
Unlike qualitative forecasting, quantitative forecasting uses mathematical models and historical data to predict future demand, making it especially relevant for retailers with access to consistent sales data. This approach is ideal for situations where patterns or trends are apparent, such as established product lines or predictable sales cycles.
Quantitative forecasting is best used when there is ample historical data and stable market conditions.
Quantitative forecasting techniques and examples
- Time series analysis: historical data is analyzed to identify patterns like seasonality or cyclic trends. A retailer may use time series analysis to anticipate spikes in sales during holidays or back-to-school seasons.
- Moving averages: a smoothing technique that calculates the average of past data over a set period to predict future demand. This method helps retailers smooth out short-term fluctuations and identify long-term trends. A clothing retailer might use moving averages to determine average monthly sales and plan stock accordingly.
- Exponential smoothing: applies more weight to recent observations, making it useful when demand patterns change rapidly. A tech retailer might use exponential smoothing to forecast demand for the latest gadgets, giving more weight to recent sales as trends evolve.
- Regression analysis: examines the relationship between demand and external factors like advertising spend or economic conditions. A retailer could use regression to predict how an increase in social media marketing will impact product demand.
- Causal models: the impact of external factors like price changes or economic indicators on demand. A retailer might use causal models to predict how a price increase in raw materials would affect product demand.
Hybrid methods: the best of both worlds
Hybrid forecasting methods combine qualitative and quantitative approaches to enhance forecast accuracy, making them particularly relevant for retailers navigating complex or uncertain markets.
By integrating both data-driven insights and expert judgment, hybrid methods provide a more comprehensive view of potential demand.
Hybrid methods are best utilized when uncertainty or rapid market changes make relying solely on historical data risky.
For example, a retailer launching a new product might not have sufficient sales data for traditional quantitative models. In this case, they can supplement market research (qualitative) with time series analysis of similar product lines (quantitative) to generate more reliable demand predictions. This integration allows retailers to balance the precision of quantitative data with the market insights provided by experts or customer feedback.
Scenario planning is another key hybrid method. Retailers develop multiple forecast scenarios based on different assumptions, such as changes in consumer behavior, economic conditions, or competitor actions.
Advanced forecasting methods: the next step in demand forecasting
Advanced forecasting methods, such as machine learning (ML), artificial intelligence (AI) and collaborative planning, forecasting and replenishment (CPFR), are transforming how retailers predict demand.
These methods are particularly valuable for retailers handling large datasets, dynamic markets, or complex supply chains, offering deeper insights and higher forecast accuracy.
Machine learning and AI leverage sophisticated algorithms to analyze vast amounts of data, identifying patterns and trends that traditional methods might miss. McKinsey found that demand forecasting augmented with AI could reduce forecasting and ordering errors by up to 50%, which in turn reduces costs by enabling more accurate inventory planning. Retailers can use AI-powered forecasting tools to assess historical sales, weather data, customer behaviors and even social media trends.
These models continuously learn and improve over time, adjusting forecasts based on new data. This makes ML and AI ideal for fast-changing industries like fashion or electronics, where customer preferences and demand fluctuate rapidly.
Collaborative planning, forecasting and replenishment involves collaboration between retailers, suppliers and other supply chain partners to share data and jointly plan for demand. By pooling data from various points in the supply chain, retailers can develop more accurate forecasts and align stock levels accordingly.
Lisa Bobb of Lightspeed retailer Squash Blossom uses a form of CPFR by sharing performance data with her vendors.
“All my vendors love that Lightspeed can generate automated reports that they get monthly. They take that and create a reorder for me. They end up getting more business out of me and it’s great because it’s my best sellers. I don’t have to guess.”
Choosing the right forecasting method
Choosing the right demand forecasting method is crucial for retail businesses, but the decision depends on several factors.
No single method fits all forecasting needs. When planning demand forecasting for your business, consider:
- Data quality: ensure your data is complete and accurate for quantitative methods. When data is lacking, supplement with qualitative approaches.
- Scalability: use advanced methods like AI if the business size and complexity require it.
- Hybrid methods: for more accuracy, integrate qualitative insights from market research or expert judgment with quantitative models.
Here are the top four key factors to consider when selecting a forecasting method.
1. Data availability
The amount and quality of data available is one of the most important considerations.
- Quantitative forecasting requires historical data, such as sales records, market trends and seasonal demand patterns. If a business has a wealth of past data, methods like time series analysis or regression analysis can be used.
- Qualitative forecasting is more suitable for businesses with limited data, such as startups or companies launching new products. Expert judgment, market research or the Delphi Method are useful when historical data is unavailable or unreliable.
2. Business size
Business size also impacts which forecasting method is appropriate.
- Small businesses with fewer products and simpler operations may benefit from qualitative or simple quantitative methods like moving averages. These methods are less resource-intensive and can still provide valuable insights for managing inventory and planning.
- Larger businesses with complex operations often require more sophisticated techniques, like quantitative forecasting augmented with machine learning or AI-driven forecasting, as they deal with a broader range of products and more data points.
3. Industry
The industry a business operates in will influence demand patterns and forecasting needs.
- Retailers in fast-changing and seasonally driven industries like fashion or technology need forecasting methods that can adapt quickly to new trends and shifting customer preferences. Hybrid models that combine qualitative insights with quantitative data work well in these cases.
- Businesses in stable industries, like grocery or home goods, may rely on more traditional quantitative models, such as time series analysis, because demand is more predictable.
4. Forecasting goals
Understanding the purpose of the forecast is critical in determining the best method.
- Short-term forecasting for inventory replenishment or staffing needs can be handled by quantitative methods like exponential smoothing or moving averages.
- Long-term forecasting, such as planning for expansion or new product launches, might require a combination of qualitative and quantitative methods. Scenario planning can provide flexibility by accounting for different market conditions or external factors.
The role of technology in stock management
Technology has revolutionized stock management, enabling businesses to streamline processes and improve accuracy—and, ultimately, improve demand forecasting.
It’s all part of one interconnected cycle. Let’s look at each part to see how.
Inventory management software
With inventory management software, retailers can automate stock tracking and get real-time insights into inventory levels, reducing the need for manual updates and minimizing errors. Handling these tasks manually is time-consuming and error prone, making demand forecasting—when you can fit it in—less reliable as a result.
Which is why inventory management software is a non-negotiable upgrade for any retailer. For businesses that operate on a perpetual inventory system, this technology continuously updates stock levels, providing real-time data that improves decision-making on restocking and demand forecasting. Periodic systems also benefit, as software can simplify and streamline the auditing process, making stock counts more efficient and accurate.
RFID (Radio Frequency Identification)
By attaching RFID tags to products, businesses can track items throughout the supply chain—and therefore get a concrete idea of just how long each step takes, helping to fine tune demand forecasting timelines.
RFID scanners automatically capture information as items move through warehouses and stores, providing real-time updates on inventory levels. This technology also improves accuracy by reducing the need for manual scanning and counting, helping businesses avoid stockouts and overstocking.
The Internet of Things (IoT)
IoT technology plays a significant role in modern inventory management by connecting devices, sensors and systems across the supply chain.
Smart shelves, for example, can monitor stock levels in real time and alert managers when products need restocking. IoT devices also provide real-time data on product conditions, such as temperature or humidity, which is essential for managing perishable goods.
This data can be fed into quantitative forecasting models to improve their accuracy.
Automation and real-time data
One of the biggest advantages of integrating technology into stock management is the automation of time-consuming tasks. Real-time data from RFID, IoT and inventory software allows businesses to make quicker and more informed decisions. Automating inventory counts, reordering processes and demand forecasting reduces human error and ensures businesses can respond quickly to market changes.
Challenges in demand forecasting
Demand forecasting is a gamechanger, but it’s not always smooth sailing. Here are some challenges to watch for (and proactively work around).
Market volatility
Unforeseen changes in market conditions, such as economic shifts, geopolitical events or sudden changes in consumer behavior, can quickly disrupt demand forecasts. A sudden recession can significantly reduce consumer spending, making previous forecasts irrelevant. New competitors or changes in customer preferences can rapidly alter demand.
To mitigate this, use flexible forecasting models and incorporate real-time data to quickly adjust predictions in response to market fluctuations.
Complex supply chains
Any disruption at one point in even a simple supply chain can cause delays throughout the entire system. Forecasting in complex supply chains with multiple suppliers and distribution channels adds another layer of difficulty.
Variability in lead times, supplier reliability and logistical constraints can disrupt product availability, making it harder to align supply with demand.
Maintain close communication with suppliers and leverage technology like collaborative planning tools to improve visibility and forecasting accuracy across the supply chain.
Data accuracy and availability
One of the biggest challenges in demand forecasting is ensuring access to accurate and timely data. Inconsistent or outdated data can lead to inaccurate forecasts, causing stock shortages or overstocking. Relying on incomplete sales data from multiple locations or channels may misrepresent actual demand. Gaps in historical data or changes in market conditions (like a sudden shift in customer preferences) can also skew forecasts.
To address these issues, invest in integrated systems that provide real-time, centralized data and regularly audit data quality for accuracy.
Automate data collection with Lightspeed Insights
Did you know Lightspeed Insights can run your reports for you?
Scheduling custom reports to run and deliver to key stakeholders daily, weekly or monthly will ensure you’re always using the most up to date data in your demand forecasting, without needing to track it down each time.
Close stock gaps with demand forecasting
By using the right forecasting methods—whether qualitative, quantitative, hybrid or advanced techniques—retailers can stay ahead of market fluctuations and make informed decisions that drive profitability.
Choosing the appropriate forecasting method and regularly updating forecasts can help businesses avoid costly stockouts or overstocking, improve operational efficiency and enhance customer satisfaction.
And having the right technology backing you up can enable more accurate forecasting through real-time data—technology like Lightspeed POS. Let’s chat about what we could do for your business.
Frequently asked questions about demand forecasting
What is the difference between demand forecasting and inventory planning?
Demand forecasting predicts future customer demand based on historical data, market trends and other factors. It helps businesses anticipate what products will be needed in the future.
Inventory planning, on the other hand, involves managing stock levels based on the demand forecast.
While demand forecasting estimates future needs, inventory planning focuses on ensuring the right products are available at the right time, optimizing stock levels and minimizing shortages or excesses.
How often should demand forecasts be updated?
Demand forecasts should be updated regularly, depending on the nature of the business and market volatility.
For most businesses, updating forecasts monthly or quarterly is typical. However, in fast-moving industries or during high-sales periods, such as holiday seasons, forecasts may need to be revised weekly or even daily.
What are some common mistakes to avoid in demand forecasting?
Common mistakes in demand forecasting include relying solely on historical data without considering market shifts, ignoring external factors like economic trends or seasonal demand and over-relying on one forecasting method.
Another key mistake is not involving different departments, such as marketing or sales, in the forecasting process.
Failing to regularly update forecasts can lead to inaccurate predictions, resulting in either stock shortages or overstocking, which can harm profitability.
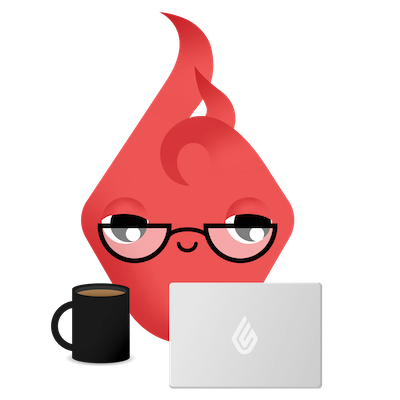
News you care about. Tips you can use.
Everything your business needs to grow, delivered straight to your inbox.